Last updated on February 6, 2025
Introduction:
Artificial Intelligence is playing a vital role in climate change improvements. In today’s era of peak industrialisation. Every other person is running after money and making profits. This kind of attitude of making more and more money and utilising than natural resources is degrading the climate. Climate change represents one of the most difficult challenges of the 21st century and it is demanding disciplined solutions. The AI has come up with the unprecedented capacities to model predict and solve environmental impact. From accelerating carbon capture research to optimising rates artificial intelligence is reshaping the climatic signs and policies. We will discover the cutting edge applications and challenges of deploying them at scale for AI driven climate solutions.
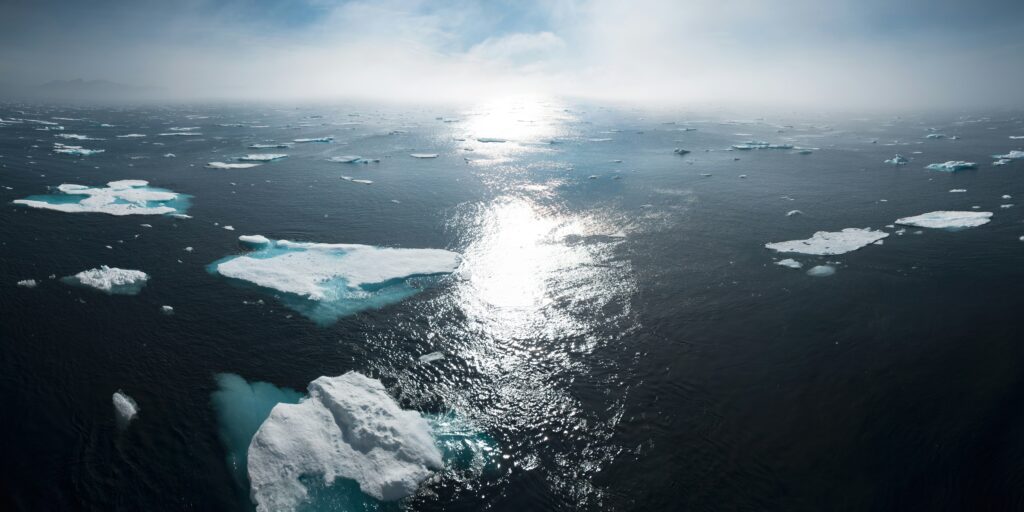
Photo by William Bossen on Unsplash
Artificial intelligence for climate modelling and prediction
Technical challenge: the traditional climate models such as the general circulation models require a huge amount of computational sources to simulate Earth’s system at a very higher resolution.
These models struggle with latency uncertainty and granularity.
Artificial intelligence solutions
Convolutional neural networks and recurrent neural networks
This processes facial data to identify patterns in the climatic variables. For example the researchers at Nvidia developed a FourCastNet which is the 4 years based neural operator which predicts extreme weather events faster 45000 Times than numerical models.
Aryans which are particularly long short term memory networks, model temporal dependencies in time series data. (Glacier melt trends, CO2 concentration trends).
Generative Adversarial Networks
Games generate synthetic climate which films gaps in the observational data sets. For example: ClimAlign framework uses GANs to align lower resolution climatic projections with higher resolution historical data improving precipitation forecast.
Transformer models
Vision Transformers process multi spectral satellite deforestation detection Google’s Arth engine leverages these transformer architecture to analyse peteabytes of Geo spatial data in real time.
Case study
European Centre for Medium Range Forecasts integrates AI with traditional models to improve hurricane predictions and trajectory. These AI based models are reducing the computational cost by 90% and maintaining 95% accuracy.
Artificial intelligence in renewable energy optimization
Technical challenge
Renewal energy sources like solar and wind are endless, requiring dynamic management to balance supply and demand.
AI Solution:
Reinforcement learning
These agents are used to optimise energy dispatch in smart grids. Deep mines Alpha grade uses proximal policy optimisation PPO to reduce wasted energy by 30% in wind farms.
Graph neural network
GNNs model power grids as graphs predicting line failure and optimising transmission. The DeepGrid framework by Stanford University minimises in decentralized solar grids.
Time series forecasting
XG boost and temporal fusion Transformers predict energy demand and renewable generation. For instance Google deepmind wind farm boosts revenue by 20% using 36 hours ahead forecast.
Case study
UK’s National grade employees AutoGrid flex, m a i platform that takes data from 10000 + iot sensors to balance grid load reducing dependency on fossil fuel pick up plants by 15%.
AI for carbon storage and carbon capture
Technical challenge while finding or identifying the optimal materials for carbon capture involves a process that is prohibitively slow. This involves testing millions of candidates.
AI Solution
Molecular Graph Network
It involves identifying 20 high performance candidates for pilot testing. The graph based models predict the adsorption capacities of MOFs. The research at MIT used crystal grass convolutional neural networks to find out from 100000 MOFs.
Bayesian optimization
This optimization technique accelerates prioritising promising material combinations. The businesses like CarbonCare use this method to optimise concrete carbonation processes.
Quantum machine learning
This machine learning procedure simulates QML algorithms at Quantum accuracy to reduce operational cost for CCS material design.
Case Study
The open catalyst project a collaboration between the meta and Carnegie Mellon, uses artificial intelligence to discover catalysts for converting carbon dioxide to ethylene. Dear models achieve DFT level accuracy with 0.001 times the computational cost.
Conclusion
AI is not an eternal solution for climate change but it can be used as a force multiplier. Bank loading physics away neural networks and reinforcement learning with Quantum computing researchers are pushing the boundaries and making what can be done possible.
But success depends on the collaboration across various disciplines such as climatic scientists AI engineers and policy makers must sit together and co-design the solutions that are robust and socially adaptable.